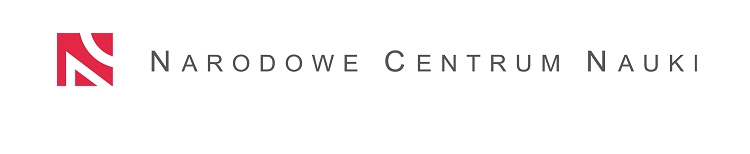
Project title: Acoustic Intelligence – towards self-supervised deep neural acoustic analysis
Project title in Polish: Acoustic Intelligence – metody samouczenia głębokich modeli neuronowych w analizie sygnałów akustycznych
Project type: Research project
Funding Institution: National Science Centre, Poland (NCN)
Program: OPUS
Project No: 2023/49/B/ST7/04100
Value: 1 189 500 PLN (276 628 EUR)
Duration: 2024 – 2028
Project team members:
dr hab. inż. Konrad Kowalczyk, prof. AGH – Principal Investigator (PI)
Motivation
Over the last couple of years, Artificial Intelligence (AI) has revolutionized the technology industry. We notice that in everyday life, as AI slowly starts to help us with a number of daily tasks. For instance, AI guards our privacy, making sure nobody unauthorized can unlock our devices while enabling us to easily access them by recognizing our face, fingerprint, or voice. Most commonly, when we say AI, we mean Deep Neural Networks (DNN) which can learn to perform extremely complex tasks by mimicking the structure of the human brain. In a nutshell, the classical way to train the DNN involves showing it millions of question-answer examples, referred to as data and labels. By feeding the questions to the DNN and comparing its answers with the ground truth, we can update the DNN so that it incrementally becomes better at solving a problem. This approach is called supervised learning and it is very effective in various isolated tasks, for which a large training dataset with appropriate labels is available.
Unfortunately, it is not straightforward to apply supervised learning to any given problem. In real-life applications, we often do not have access to reference content in general, which prevents us from using the supervised learning framework. Partially, this is because the labels have to be prepared with a specific purpose in mind, which may just not fully meet our needs for a differently defined task. Secondly, since labeling commonly has to be done manually, it is often not feasible in practice. Another issue concerns performance degradation with out-of-domain data, which happens when the training data is substantially different from the target domain. That can be the case when DNN is trained to understand speech using recordings made with a professional microphone in studio conditions, and then it processes audio from a smartphone microphone on a busy street.
Project goal
In the Acoustic Intelligence project, we direct our focus towards Self-Supervised Learning (SSL) for audio applications. Contrary to conventional learning, the supervision within the SSL is supposed to be induced from the unlabeled data itself, by capturing its structure. Besides leveraging the label-dependency problem, SSL enables to train systems on orders of magnitude more data. Thus, the models can learn to distinguish more subtle patterns, which increases their robustness, compared to fully supervised cases. This concept, so far mostly investigated in the fields of Computer Vision and Natural Language Processing, has potential to revolutionize research in the acoustic domain. We aim to expand current knowledge with research on formulation of novel cost functions, derivation of mathematical models along with corresponding selfsupervised training procedures, design and preparation of appropriate experimental evaluations.
The main goal of the Acoustic Intelligence project is to introduce novel Universal Audio Representation (UAR), Universal Acoustic Analysis (UAA), and Universal Constituent Audio Signal Enhancement (UCASE) to enable creation of stand-alone intelligent machines that can autonomously learn to improve their performance in a broad range of audio-related tasks, even in unseen acoustic test conditions. Among other, we aim to establish a new state-of-the-art with self-supervised acoustic signal enhancement and acoustic scene analysis.